- Resume Examples
- Machine Learning Engineer
4 Machine Learning Engineer Resume Examples & Writing Guide
Improve your machine learning engineer resume with 4 real examples and a detailed writing guide. Learn what to include and how to structure your resume to impress hiring managers and increase your chances of landing an interview. By implementing these proven tips and techniques, you can create a resume that effectively showcases your skills and experience.
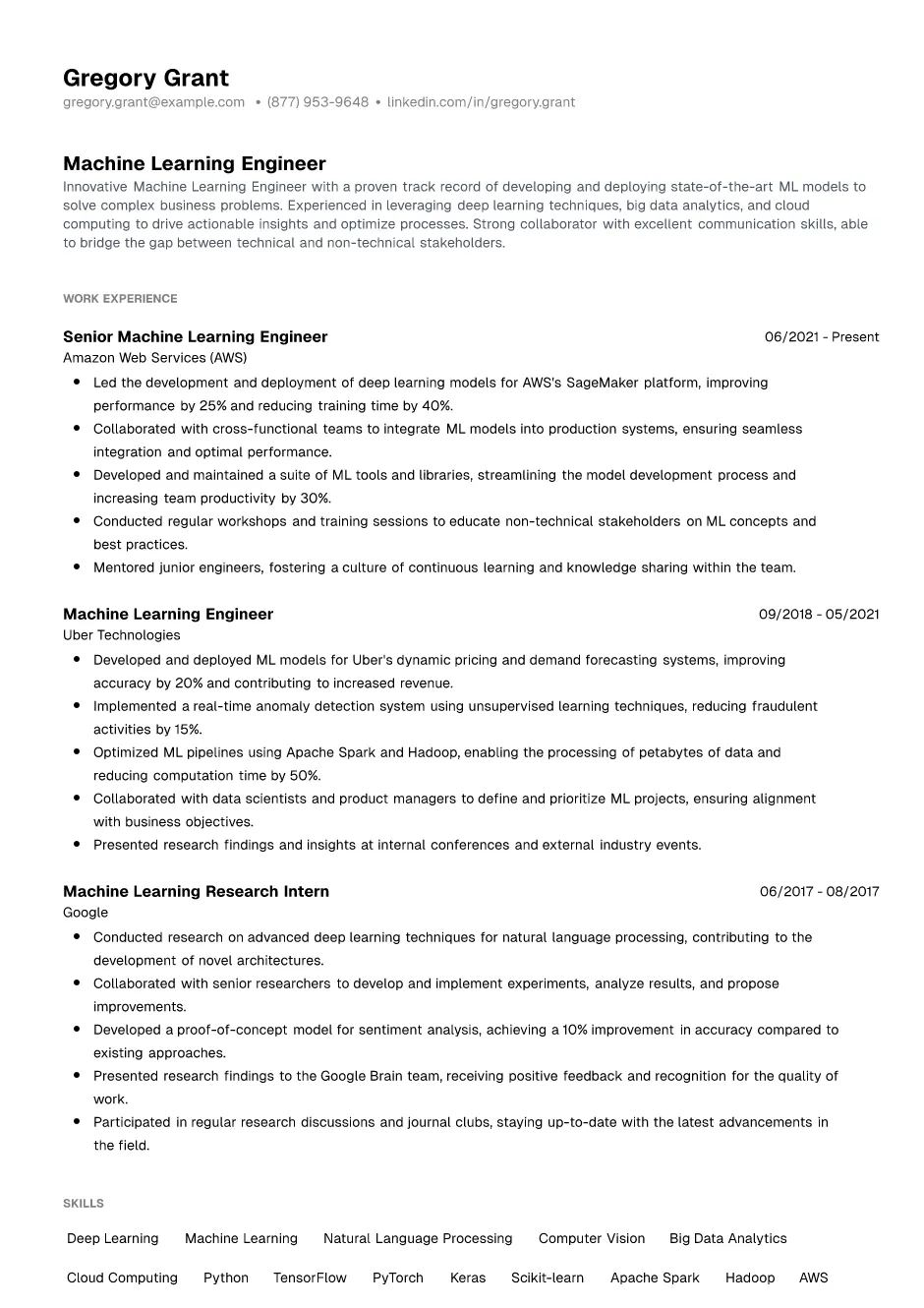
In the fast-paced field of machine learning, a great resume is essential to land the job you want. With many qualified candidates competing for open positions, your resume needs to grab the attention of hiring managers and showcase your top skills and experience.
But what does a winning machine learning resume look like? How can you summarize your qualifications, projects, and achievements in a way that quickly conveys your value to potential employers?
In this article, you'll find four real-world examples of machine learning engineer resumes that get results. We'll break down what these resumes do well and explain how you can apply their strategies to your own job search. You'll also get step-by-step guidance on structuring your resume, choosing the right keywords, highlighting your most relevant projects, and avoiding common mistakes.
Whether you're an experienced machine learning pro or just starting your career, these tips and examples will help you create a resume that rises to the top of the stack and boosts your chances of scoring an interview. Let's dive in and see how to make your machine learning resume shine.
Common Responsibilities Listed on Machine Learning Engineer Resumes
- Developing and implementing machine learning models and algorithms
- Collecting, cleaning, and preprocessing data for model training
- Selecting and engineering appropriate features for model input
- Evaluating and optimizing model performance using statistical techniques
- Building and deploying scalable machine learning systems and pipelines
- Collaborating with cross-functional teams, including data scientists and software engineers
- Staying up-to-date with the latest machine learning techniques and tools
- Communicating complex technical concepts to non-technical stakeholders
- Identifying and addressing ethical considerations in machine learning applications
How to write a Resume Summary
The Significance of a Summary/Objective in Your Resume
The summary or objective section of your resume serves as an introductory handshake with potential employers. It's your initial chance to express who you are, what you bring to the table, and how you can contribute to the organization's growth. It's quite much like the abstract of a research paper, providing a concise overview of what to expect in the full document.
As a Machine Learning Engineer, you deal with complex algorithms and intricate data sets, it's all about simplifying that complexity into easily understandable terms when it comes to your resume summary. Your technical expertise is unquestionable, but how you portray that expertise to prospective employers is what distinguishes you from the crowd. This is neither the place to list every coding language you know nor an opportunity to delve into detailed aspects of previous projects.
Optimize for Clarity
Simplicity and clarity form the backbone of a memorable resume summary. The use of specific, straightforward language can help potential employers quickly grasp your qualifications and achievements. Keep in mind that a hiring manager is not always a technical expert in your field - they should still be able to understand what you're mentioning and why it's significant.
Highlight Your Expertise and Authoritativeness
As a Machine Learning Engineer, any potential employer would want to know about your understanding and proficiency in machine learning concepts and algorithms, programming languages, and related tools you're proficient with. However, merely mentioning them is not enough. You've got to demonstrate the impact of your skills in real-world scenarios by referring to notable accomplishments or highlighting how your expertise was used in prior roles. In addition, you should underline your problem-solving skills, creativity, and ability to work in a team, blend technical skills seamlessly with your interpersonal skills.
Establish Your Trustworthiness
Trustworthiness can be expressed in your resume summary through evidence of your reliability and consistency. For instance, you could mention long-term roles you've held, prominent projects you've seen through to completion, or the learning curve you've undergone to fine-tune your expertise.
Balance Between Brevity and Details
While you want to keep your resume summary concise, reducing it down to a vague or general statement will likewise not serve you well. Aim for a balance. Let every word serve a purpose and provide value, focus on quality over quantity.
Addressing these aspects in your resume summary will not only provide a lucid snapshot of your qualifications and career trajectory but also paint a vivid picture of the unique professional you are. After all, a resume is not just a list of qualifications and experiences; it is a testament to your professional journey.
Strong Summaries
- Results-oriented Machine Learning Engineer with over five years of experience managing sophisticated algorithmic and statistical methodology to assist businesses. Expert in Machine Learning theories and able to translate them into applicable strategies.
- Driven Machine Learning Engineer, proficient in predictive modelling, data processing, and algorithm development. Proven ability to drive efficiencies through automation, by leveraging skills in Python and TensorFlow.
- Experienced machine learning engineer with a deep understanding of neural networks, decision trees, and regression models. Successfully increased model accuracy by 20% in my previous role and eager to bring these results to new projects.
- Detail-oriented Machine Learning Engineer with a passion for accuracy and precision. Noted for designing sleek predictive models and having a keen eye for data analysis. Skilled in Python, TensorFlow and Keras.
- Innovative Machine Learning Engineer with a proven track record of identifying trends and insights by leveraging machine learning. Recognized for improving analytical capabilities and increasing operational efficiency.
Why these are strong?
The above examples are good practices as they demonstrate a variety of essential components that an engaging and effective Professional Summary for a Machine Learning Engineer's resume should contain. These elements include mentioning specific technical skills, such as proficiency in Python, TensorFlow, knowledge of neural networks, decision trees, regression models, etc. Showcasing achievements or quantitative results – like increasing model accuracy or improving operational efficiency – can also set a candidate apart. Lastly, summarizing their experience or approach in a unique manner enhances their personal brand and demonstrates their passion for their work.
Weak Summaries
- Professional Machine Learning Engineer who likes cats and long walks on the beach. Have some experience in machine learning and computer science. Kind of good with numbers.
- I'm a Machine Learning Engineer and I have done some things related to this field. Seeking for a role where I won't be bothered too much and can work on my own things. I know Python and R and some other things.
- Being a Machine Learning Engineer has always been my big dream. After finishing my degree in psychology, I did a 2 weeks online course on machine learning and now here I am. Looking forward to work in a big company with lots of benefits.
- Machine Learning Engineer ready to work. Has experience. Knows things. If picked I promise to work hard and not cause problems.
Why these are weak?
All of the above examples are vague, unprofessional, and don't present a focused career objective. The first example brings up personal hobbies which are irrelevant in the professional summary. The second example is unenthusiastic, and fails to mention any key qualifications or achievements. The third example doesn't go into specifics about experience or highlight core logistic skills, and it mentions a career shift as if it was irrelevant. The fourth example is too brief and generic, providing no substance or specifics about the candidate's skills or experience. Use the professional summary to highlight key qualifications, achievements, and a clear career objective.
Showcase your Work Experience
The Work Experience section of your resume is your opportunity to showcase the skills you've acquired and demonstrate how you've applied them in real-world scenarios. As a Machine Learning Engineer, this aspect of your resume becomes even more vital, as employers are interested in understanding exactly what you can offer in this highly specialized field.
Start with your most recent position
First and foremost, always list your work experience in reverse chronological order, commencing with your most recent role. It's helpful for prospective employers to know where you are currently in your career progression, or where you were when you last worked. This awareness can play a significant role in their hiring decision.
Underscore your responsibilities
In each job description, it's important to highlight not just your key responsibilities, but also the specific tasks or projects you worked on. As a Machine Learning Engineer, theatre of your skills and abilities revolves around data analysis, creating algorithms, and machine learning model deployments amongst other things. Bring these aspects to the fore, helping the reader gain a deeper understanding of your role function.
Quantify your achievements and impact using concrete numbers, metrics, and percentages to demonstrate the value you brought to your previous roles.
Highlight your achievements
Improve the impact of your Work Experience section by focusing not just on what you did, but also on the positive outcomes that arose due to your involvement. Did the machine learning model you developed improve efficiency or accuracy? How did your role influence the projects or the company's overall success? Equip your prospective employer with quantifiable data that accentuates your ability to drive effective results.
Maintain precision
It's pivotal to avoid vague or generic phrases. Be precise about the technology, processes, and systems you've engaged with. As a Machine Learning Engineer, your understanding and usage of various programming languages, libraries, and ML algorithms become key determinants of your fitment for the role.
Cater to the job requirements
Every role differs, so does its requirements. Therefore, make sure to tailor your resume to suitably match the specific job post. Recognize the keywords and abilities cited in the position description and reflect pertaining aspects from your own experience. This precision can favourably set you apart.
Remember, your resume is your marketing tool - it is passing 'you' around. However, avoid a mere listing of your experiences or skills, aim to tell a coherent story, stitching together your learning and growth. A well-devised work experience section can make you more relatable and reassure the employers of your potential fit within their targeted role. Hence, invest time and thought into it, ensuring it helps you build a substantial connection with your prospective employer.
Strong Experiences
- Developed and implemented predictive models using Python and machine learning libraries, resulting in a 20% improvement in predictive accuracy.
- Led a team in the design and execution of an AI-driven product recommendation system, leading to a 15% increase in sales.
- Implemented automated data cleaning and preprocessing, improving data quality and reducing manual data management workload by 30%.
- Presented research on machine learning algorithms at prestigious conferences, demonstrating company's commitment to cutting-edge technology.
Why these are strong?
The above examples are considered good as they provide measurable achievements instead of just stating duties. They show a proactive approach toward improving performance and contributing to the business's success. Furthermore, they provide specific technical context (Python, machine learning libraries, AI-driven system) that indicates a thorough understanding of job requirements and ability to apply this knowledge on practical tasks. Also, they demonstrate leadership and communication skills which are critical for any position.
Weak Experiences
- - Worked on stuff related to machine learning.
- - Used Python and some other tools to play with data.
- - Don't remember what exactly I did, but it was something important.
- - Did project on machine learning. It was fun.
- - Participated in some meetings and helped people to understand machine learning.
Why these are weak?
These examples lack specificity, professionalism, and do not convey the skills or accomplishments of the candidate effectively. First, phrases like 'worked on stuff' or 'play with data' are vague and fail to explain the nature of the work or responsibilities. This can make it impossible for hiring managers to appreciate the candidate's role and achievements. Second, statements like 'don't remember what exactly I did' give off a lack of interest or commitment in the task, which is a major red flag for employers. Thirdly, casual language such as 'it was fun' is unprofessional and fail to present the candidate in a serious light. Finally, 'Participated in some meetings' is too generic and tells nothing about the candidate's contribution or the value they brought to the project or team.
Skills, Keywords & ATS Tips
Building a resume that stands out is crucial to winding your way into your dream job. Major focus should be given to skills, both hard and soft, primarily because hiring managers are interested in these. For a machine learning engineer, it's not just about what you know technically (hard skills), but also how well you handle problems and work with others (soft skills). Skillfully adding this information to your resume can also significantly improve your chances with an Applicant Tracking System (ATS).
Hard Skills in a Machine Learning Engineer Resume
Hard skills are quantifiable, teachable abilities that are job-specific. As a machine learning engineer, your hard skills could include programming languages like Python or R, experience with machine learning frameworks like TensorFlow or PyTorch, or knowledge of cloud platforms such as AWS or Google Cloud.
The technical nature of your role means that these hard skills are vital and should be prominently featured on your resume. When creating or editing the skills section, match them with the job description if they align with your skillset. This synchronization helps you get past the ATS which employers use to screen and sort through resumes based on matches with job requirements.
Soft Skills in a Machine Learning Engineer Resume
Soft skills are not job-specific but they are crucial for a well-rounded professional profile. They include communication, teamwork, problem solving or attention to detail.
In the context of a machine learning engineer position, your soft skills demonstrate to the employer your ability to work as a part of team, communicate complex ideas or ensure the precision of your work. They prove that you're not just a technical robot but a human who can thrive in a dynamic work environment.
Remember, while hard skills show your machine learning abilities, it's your soft skills that differentiate you from others who have similar hard skills.
Keywords, ATS and Matching Skills
An ATS is a software tool that many hiring managers use for the initial resume screening process. It scans resumes for keywords—usually those listed in the job description—before human eyes ever see them. This means that even if you're the best candidate for the job, you could be overlooked if your resume doesn't contain the right keywords.
In the skills section of your resume, ensure you incorporate hard and soft skills that match those in the job description. This doesn't mean filling your resume with keywords for the sake of it. Rather, it's about a thoughtful representation of your abilities that correspond with the job requirements. This will not only get you past the ATS but also show the prospective employer that you have what they're looking for.
In sum, giving due diligence to hard and soft skills and understanding the role of keywords and ATS are integral in creating a standout machine learning engineer resume. Always remember, your skills and how you present them can be the differentiating factor in getting you that interview call.
Top Hard & Soft Skills for Full Stack Developers
Hard Skills
Soft Skills
Top Action Verbs
Use action verbs to highlight achievements and responsibilities on your resume.
Education & Certifications
As a Machine Learning Engineer, showcasing your education and certificates on your resume is significant. Start this section by listing your highest degree first, then proceed in reverse chronological order. Include the title of each degree, the institution you attended, and the dates of graduation. For certificates, mention the specifics like the name of the certificate, who issued it, and when. This diligent cataloging can highlight your skill set and the level of dedication you have for constant learning in your field.
Some of the most important certifications for Machine Learning Engineers
Demonstrate your proficiency in using TensorFlow to implement machine learning models.
Validate your ability to design, implement, deploy, and maintain machine learning solutions on AWS.
Demonstrate your expertise in analytics by earning the CAP credential.
Learn key data science tools and earn a certificate from IBM.
Master deep learning techniques and earn a specialization certificate.
Resume FAQs for Machine Learning Engineers
What is the ideal resume format for a Machine Learning Engineer?
The most recommended resume format for a Machine Learning Engineer is the reverse-chronological format. This format highlights your work experience and achievements in a clear and organized manner, which is essential for technical roles like Machine Learning Engineering.
How long should a Machine Learning Engineer resume be?
A Machine Learning Engineer resume should typically be one page long for candidates with less than 10 years of experience, and no more than two pages for those with more extensive experience. Concise and focused resumes are preferred in the tech industry.
What technical skills should be included in a Machine Learning Engineer resume?
Some essential technical skills to include in a Machine Learning Engineer resume are programming languages (Python, R, Java, C++), machine learning frameworks (TensorFlow, PyTorch, Scikit-learn), data manipulation (SQL, Pandas, NumPy), and cloud platforms (AWS, GCP, Azure). Additionally, include any specialized skills like deep learning, natural language processing, or computer vision.
How can I highlight my machine learning projects on my resume?
When highlighting machine learning projects on your resume, focus on the problem you solved, the techniques and tools you used, and the quantifiable results or impact of your work. Provide brief but clear descriptions of the projects, and consider including links to GitHub repositories or online portfolios.
Should I include personal projects on my Machine Learning Engineer resume?
Absolutely! Personal projects are an excellent way to demonstrate your passion for machine learning, as well as your ability to learn and apply new techniques independently. Be sure to highlight the skills you utilized and the outcomes of these projects.
How can I make my Machine Learning Engineer resume stand out?
To make your Machine Learning Engineer resume stand out, tailor it to the specific job you're applying for by using relevant keywords from the job description. Additionally, quantify your achievements with metrics, showcase your problem-solving abilities through project descriptions, and highlight any publications, presentations, or industry recognition you've received.
A Machine Learning Engineer develops and deploys artificial intelligence systems. Key responsibilities include gathering and preprocessing data, building predictive models, evaluating model accuracy, and optimizing performance. When crafting a resume, emphasize technical skills like Python, experience with frameworks like TensorFlow, and strong quantitative abilities. Highlight relevant projects, internships, and use keywords from job listings to make your resume stand out.
Innovative Machine Learning Engineer with a proven track record of developing and deploying state-of-the-art ML models to solve complex business problems. Experienced in leveraging deep learning techniques, big data analytics, and cloud computing to drive actionable insights and optimize processes. Strong collaborator with excellent communication skills, able to bridge the gap between technical and non-technical stakeholders.
- Led the development and deployment of deep learning models for AWS's SageMaker platform, improving performance by 25% and reducing training time by 40%.
- Collaborated with cross-functional teams to integrate ML models into production systems, ensuring seamless integration and optimal performance.
- Developed and maintained a suite of ML tools and libraries, streamlining the model development process and increasing team productivity by 30%.
- Conducted regular workshops and training sessions to educate non-technical stakeholders on ML concepts and best practices.
- Mentored junior engineers, fostering a culture of continuous learning and knowledge sharing within the team.
- Developed and deployed ML models for Uber's dynamic pricing and demand forecasting systems, improving accuracy by 20% and contributing to increased revenue.
- Implemented a real-time anomaly detection system using unsupervised learning techniques, reducing fraudulent activities by 15%.
- Optimized ML pipelines using Apache Spark and Hadoop, enabling the processing of petabytes of data and reducing computation time by 50%.
- Collaborated with data scientists and product managers to define and prioritize ML projects, ensuring alignment with business objectives.
- Presented research findings and insights at internal conferences and external industry events.
- Conducted research on advanced deep learning techniques for natural language processing, contributing to the development of novel architectures.
- Collaborated with senior researchers to develop and implement experiments, analyze results, and propose improvements.
- Developed a proof-of-concept model for sentiment analysis, achieving a 10% improvement in accuracy compared to existing approaches.
- Presented research findings to the Google Brain team, receiving positive feedback and recognition for the quality of work.
- Participated in regular research discussions and journal clubs, staying up-to-date with the latest advancements in the field.
A Senior Machine Learning Engineer is responsible for developing and deploying cutting-edge ML models that drive innovation. They leverage expertise in algorithms, data structures, and coding to architect robust data pipelines and scalable model infrastructures. With a master's degree and over 5 years of experience, they lead teams in building and optimizing complex ML systems. When crafting a resume, highlight technical proficiencies like Python, TensorFlow, and data wrangling. Quantify the impact of your ML projects, showcasing problem-solving abilities. Emphasize leadership in collaborative model development and deployment across cross-functional teams. Demonstrate a track record of delivering predictive solutions that create business value.
Innovative Senior Machine Learning Engineer with a proven track record of delivering cutting-edge solutions that drive business growth. Adept at leveraging deep learning, computer vision, and natural language processing techniques to develop intelligent systems. Collaborates effectively with cross-functional teams to align technical solutions with strategic objectives.
- Led a team of 5 engineers to develop and deploy deep learning models for Amazon's product recommendation engine, improving click-through rates by 18%.
- Implemented a novel transfer learning approach to rapidly train models for new product categories, reducing development time by 40%.
- Designed and built a distributed machine learning pipeline using Apache Spark and Amazon SageMaker, enabling the processing of petabytes of data.
- Conducted regular code reviews and mentored junior engineers, fostering a culture of continuous learning and improvement.
- Presented research findings at the annual Amazon Machine Learning Conference, showcasing the team's innovative work to over 1,000 attendees.
- Developed machine learning models to optimize Uber's dynamic pricing algorithm, resulting in a 12% increase in revenue.
- Built and maintained a deep learning framework for real-time object detection in self-driving vehicles, improving safety and reducing accidents.
- Collaborated with the UX team to design and implement a personalized user experience based on machine learning insights, increasing user engagement by 25%.
- Created and delivered training sessions on machine learning best practices for engineers and data scientists across the organization.
- Contributed to Uber's open-source projects, including the development of a popular data visualization library for machine learning models.
- Conducted research on advanced deep learning architectures for natural language understanding, leading to a 20% improvement in model accuracy.
- Developed a novel approach for few-shot learning, enabling models to learn from limited training data and adapt quickly to new tasks.
- Published 3 papers in top-tier machine learning conferences, including NeurIPS and ICML.
- Collaborated with IBM product teams to transfer research findings into production-ready solutions, driving innovation across the organization.
- Mentored PhD interns and guided their research projects, helping them develop valuable skills and contribute to the team's success.
An entry-level machine learning engineer designs, trains, and deploys machine learning models to solve real-world problems. Responsibilities include data preprocessing, model selection, hyperparameter tuning, and integration into applications. Qualifications are a bachelor's in computer science or related field, proficiency in Python and ML frameworks like TensorFlow, and basic knowledge of algorithms and statistics. For the resume, highlight relevant coursework, projects utilizing ML techniques, internships applying ML solutions, and technical skills. Clearly describe your ML experience with concise, quantifiable details. Keep it well-organized and focused on your key ML qualifications.
Passionate and innovative Machine Learning Engineer with a strong foundation in data analysis, algorithm development, and model deployment. Excels at collaborating with cross-functional teams to deliver cutting-edge solutions that drive business value. Committed to continuous learning and staying at the forefront of machine learning advancements.
- Developed and implemented deep learning models for natural language processing tasks, improving accuracy by 15%.
- Collaborated with the research team to explore novel approaches to unsupervised learning, resulting in a new algorithm that reduced training time by 20%.
- Conducted data preprocessing and feature engineering on large datasets, enhancing model performance and scalability.
- Participated in code reviews and contributed to the development of best practices for machine learning projects within the organization.
- Presented findings and insights to senior leadership, demonstrating the potential impact of machine learning on key business objectives.
- Assisted in the research and development of advanced machine learning techniques for computer vision applications.
- Implemented and optimized algorithms for object detection and image segmentation, improving accuracy by 10%.
- Conducted extensive literature reviews and contributed to the writing of research papers and grant proposals.
- Collaborated with a team of graduate students and faculty members to explore new directions in deep learning research.
- Maintained and updated the lab's codebase and documentation, ensuring reproducibility and facilitating knowledge sharing.
- Conducted exploratory data analysis and feature engineering on large datasets from Amazon's product catalog.
- Developed machine learning models to improve product recommendation accuracy, resulting in a 5% increase in click-through rates.
- Collaborated with cross-functional teams to identify opportunities for leveraging machine learning in various business units.
- Created data visualizations and dashboards to communicate insights and model performance to stakeholders.
- Participated in workshops and training sessions to expand knowledge of big data technologies and best practices in data science.
Deep Learning Engineers develop and optimize neural networks and algorithms for machine learning models, gathering and preprocessing data, training models, and deploying them to production systems. When writing a resume, highlight your education in computer science/related fields, relevant work experience, projects involving deep learning frameworks (TensorFlow, PyTorch), programming skills (Python, C++, SQL), and knowledge of machine learning concepts.
Innovative Deep Learning Engineer with a proven track record of developing cutting-edge neural network architectures and optimizing model performance. Passionate about leveraging the power of deep learning to solve complex real-world problems and drive technological advancements. Skilled in Python, TensorFlow, PyTorch, and deploying models in production environments.
- Developed and implemented advanced deep learning models for AWS's Natural Language Processing (NLP) services, improving accuracy by 25%.
- Optimized model training pipelines using distributed computing techniques, reducing training time by 40%.
- Collaborated with cross-functional teams to integrate deep learning models into production systems, serving millions of users.
- Mentored junior engineers and conducted workshops on deep learning best practices, fostering a culture of innovation and knowledge sharing.
- Contributed to the development of AWS's open-source deep learning framework, increasing adoption by 30%.
- Conducted cutting-edge research on generative adversarial networks (GANs), resulting in a novel architecture that improved image generation quality by 30%.
- Developed deep reinforcement learning algorithms for robotic control, enabling robots to learn complex tasks 50% faster.
- Published research findings in top-tier machine learning conferences, including NeurIPS and ICML.
- Collaborated with Google's product teams to apply research findings to real-world applications, such as image search and translation.
- Presented research at internal and external events, effectively communicating complex technical concepts to diverse audiences.
- Developed deep learning models for IBM Watson's cognitive services, improving sentiment analysis accuracy by 20%.
- Implemented transfer learning techniques to reduce model training time by 30% and improve generalization.
- Optimized model deployments using Docker and Kubernetes, ensuring high availability and scalability.
- Collaborated with product managers and UX designers to create intuitive interfaces for Watson's AI-powered applications.
- Conducted code reviews and provided technical guidance to junior team members, promoting best practices and code quality.